Your basket is currently empty!
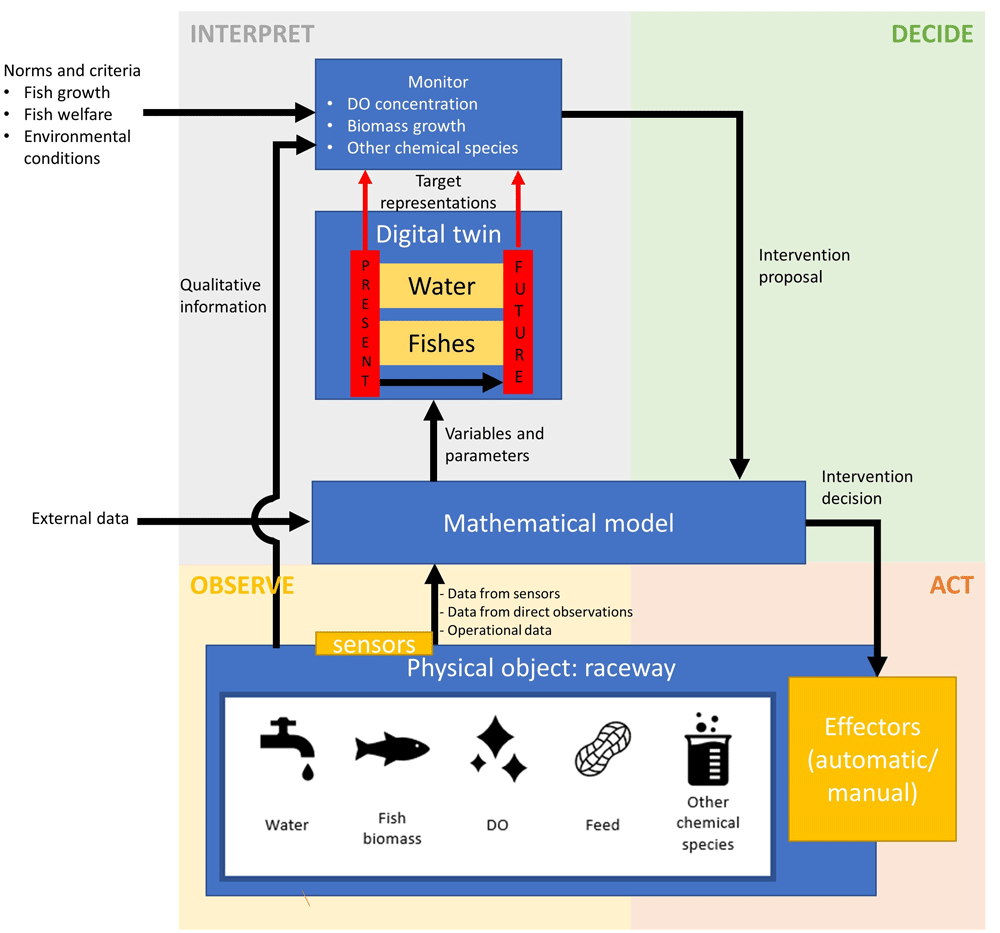
Implementing the Anymal Anytime Concept with Digital Twin Technology
Research Proposal
Introduction
The Anymal Anytime concept represents a transformative approach in sustainable aquaculture. This innovative system addresses critical challenges by enabling year-round fish production through advanced Closed Recirculating Aquaculture Systems (CRAS). These systems significantly outperform traditional fish farming methods. Up to 100 times greater output while consuming less than 5% of the water required. CRAS functions by continuously filtering and reusing water, which minimizes environmental impact while ensuring scalability for global applications.
Aquaculture faces several challenges. These include inefficiencies in water and energy usage. Additionally, there is variability in fish growth rates and the inability to predict operational disruptions accurately. To tackle these challenges, this research proposes integrating Digital Twin technology. A Digital Twin is a real-time virtual representation of physical systems. It enables operators to simulate fish growth, environmental conditions, and operational parameters. By leveraging actionable insights provided by these simulations, aquaculture facilities can optimize operations, predict outcomes, and effectively address challenges. The integration of CRAS and Digital Twin technology provides a foundation for creating resilient, efficient, and sustainable aquaculture systems.
Research Objectives
This study aims to integrate Digital Twin technology with the Anymal Anytime concept to revolutionize sustainable aquaculture. Each objective is designed to address critical components of this integration:
- Define a Digital Twin Framework: Develop a conceptual model tailored to the Anymal Anytime system. This model will align with the system’s operational goals. It will integrate sustainability metrics. Key performance indicators (KPIs) will be incorporated, including feed conversion ratio (FCR), water quality, and energy efficiency.
- Evaluate Current Technologies: Conduct a comprehensive review of available tools, like IoT sensors and data analytics platforms. This evaluation will assess their readiness and potential for integration into a Digital Twin ecosystem. It will focus on bridging existing technology gaps.
- Tackle Knowledge Gaps: Identify and analyze gaps in data acquisition, model integration, and operational scalability. The study will propose solutions, including hybrid modeling approaches, to solve these gaps effectively.
- Show Feasibility through Case Studies: Confirm the practical application of Digital Twins in aquaculture through real-world scenarios. These case studies will highlight pathways for adoption and emphasize the operational and environmental benefits of the proposed system.
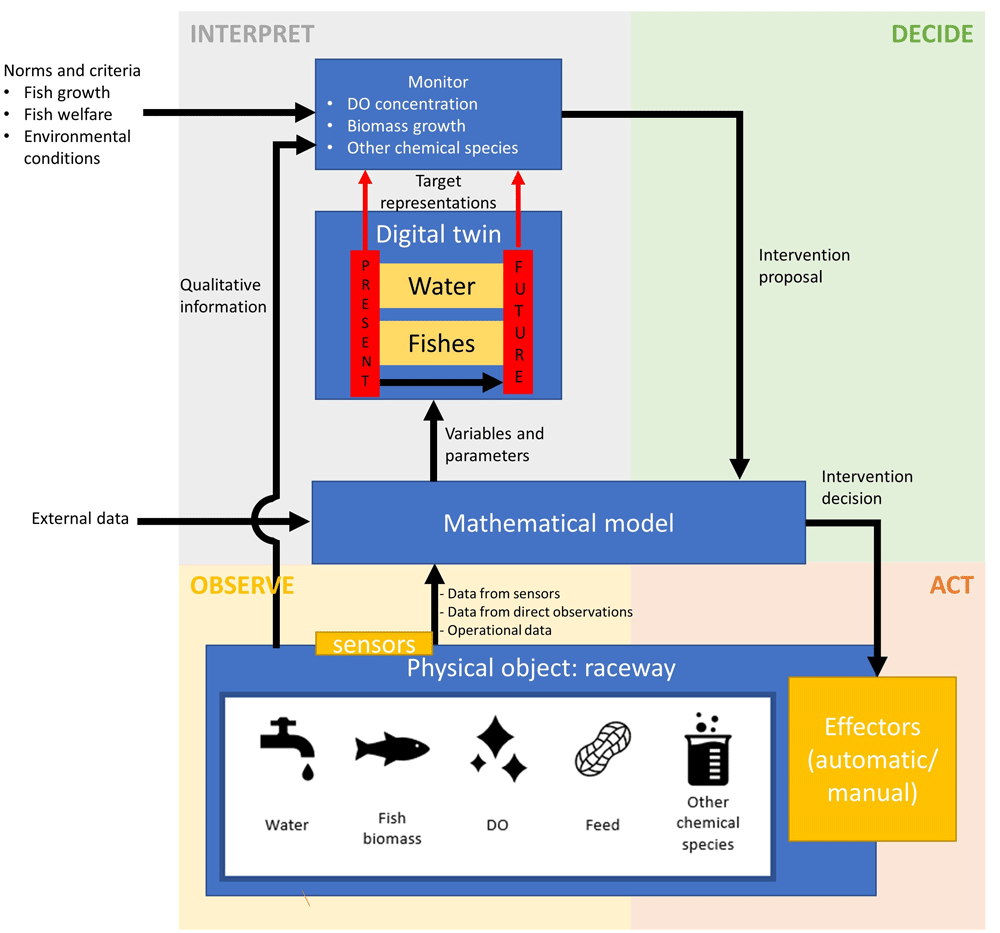
Methodology
This research will employ a structured approach to develop, validate, and refine the integration of Digital Twin technology with CRAS:
- Concept Development: The initial phase will involve reviewing literature on Digital Twin applications in other industries. Examples include manufacturing and healthcare. These systems have shown significant benefits in these fields. By studying these applications, this research will adapt relevant strategies to aquaculture. This phase will define the system boundaries. It will also identify key performance indicators (KPIs) for the Anymal Anytime system. Examples of these KPIs include feed conversion ratio (FCR), water quality, and energy efficiency. These KPIs will ensure that the model aligns with real-world operational goals. They will be tailored to the unique challenges of aquaculture.
- Technology Assessment: The study will evaluate available tools. Examples include IoT sensors for water quality, AI-based growth prediction models, and software platforms for data integration. Gaps in technology will be identified and prioritized, forming the basis for future research and development.
- Model Development: A prototype Digital Twin will be created for a single Anymal CRAS unit. This prototype will simulate key parameters such as fish growth, nutrient cycles, and system maintenance requirements. The prototype will serve as a proof of concept for the integration of Digital Twin technology.
- Case Study Implementation: The prototype will be deployed in a controlled environment. It will collect real-time data on fish health. The system also monitors water quality and operational performance. We will test scenarios such as varying feed types. We will also respond to equipment failures to validate the model’s effectiveness under diverse conditions.
- Analysis and Validation: The process involves comparing Digital Twin predictions with actual system performance. This will help to assess accuracy and reliability. Benefits such as reduced feed waste, improved growth rates, and lower operational costs will be quantified. Feedback from case studies will be used to refine the model further.
Expected Outcomes
The research is expected to deliver several impactful outcomes that can reshape aquaculture practices:
- Framework Development: A functional and scalable framework for implementing Digital Twins in aquaculture tailored to the Anymal Anytime system. Success will be evaluated by the model’s ability to simulate real-world parameters accurately and optimize operations in controlled environments.
- Technology Integration: Identification and seamless integration of the most critical technologies into aquaculture systems. Key metrics for success will include reductions in water usage, improved energy efficiency, and enhanced data processing capabilities.
- Case Study Validation: Proof-of-concept case studies demonstrating optimized performance, reduced costs, and improved sustainability in Anymal Anytime systems. Validation will rely on performance benchmarks established during the initial phases of research.
- Strategic Recommendations: Actionable insights for scaling the Digital Twin model across larger systems, different species, and diverse operational environments. Recommendations will prioritize measurable improvements in operational efficiency and sustainability.
Identified Knowledge Gaps
The concept of Digital Twins in aquaculture is promising. However, several knowledge gaps need to be addressed to realize its full potential. This section highlights both the challenges and potential solutions:
- Integration of Models: Many existing models operate independently, focusing on either biological, structural, or environmental dynamics. Bridging these domains into a unified framework is essential for creating a holistic Digital Twin. Collaborations between biologists, engineers, and data scientists could provide the interdisciplinary expertise required for integration.
- Hybrid Modeling: Current knowledge-based models lack sufficient fidelity to address complex interactions in aquaculture systems. Combining these models with data-driven approaches through hybrid modeling techniques can temporarily fill gaps. For example, machine learning algorithms can complement traditional models by predicting unobserved variables.
- Data Accessibility and Quality: Ensuring real-time access to accurate and comprehensive sensor data is essential. Challenges include sensor calibration, data standardization, and handling large datasets for analysis. Improved sensor technology and cloud-based data storage solutions could address these challenges.
- Scalability: Scaling Digital Twin solutions from pilot projects to full commercial systems remains a challenge. This difficulty is due to cost, computational resources, and integration with existing setups. Modular approaches to system design may help mitigate scalability issues.
- Predictive vs. Autonomous Systems: Current Digital Twins focus primarily on predictive capabilities. Moving to autonomous systems will need innovations in AI, robotics, and decision-making algorithms. These innovations are necessary for tasks such as feeding, cleaning, and monitoring. Pilot projects involving semi-autonomous systems serve as a stepping stone.
- Matching Supply with Demand: Accurately forecasting demand and aligning it with production capacities is challenging. The Anymal Anytime concept’s flexibility through multi-parallel processing (MPP) enables rapid adjustments to delivery schedules and production outputs. This adaptability can address short-term demand surges, long-term market planning, and seasonal variations, ensuring reliability and efficiency.
Significance of the Study
Integrating Digital Twin technology with the Anymal Anytime concept offers transformative potential for aquaculture and global sustainability. This integration:
- Enable real-time, data-driven decision-making to optimize fish growth, feed utilization, and resource management.
- Achieve significant reductions in water and energy consumption, setting a benchmark for eco-friendly aquaculture practices.
- Adapt dynamically to market demands, climate variability, and operational challenges through robust monitoring and predictive capabilities.
- Scale sustainably to meet the rising global demand for seafood. This approach reduces pressure on overfished natural stocks. It also contributes to food security.
By addressing these areas, this research aligns with broader goals of sustainable development. It supports responsible aquaculture practices. This helps pave the way for a more resilient and efficient industry.
Conclusion
This research will provide a roadmap for integrating advanced technology into sustainable aquaculture systems. By implementing Digital Twin technology, the Anymal Anytime concept can achieve its full potential. It offers a global solution for year-round, environmentally friendly fish production.
Appendix
Initial Research Questions, valid in CRAS too.
- Is it possible to define a Digital Twin concept for aquaculture applications that enables the exploitation of the advantages of this emerging technology?
- Which tools and components needed to this end are already available through the current state-of-the-art within aquaculture technology?
- What are the major knowledge gaps we need to fill to realize Digital Twins in this field?
- Is it possible through concrete case studies to highlight the way onward toward achieving this goal?
References
Leave a Reply